5.3. ML Gone Wrong¶
But just because you can, doesn’t mean you should.
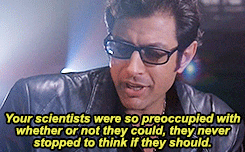
Note
Notice the company names below! These are the creme de la creme of tech firms!
ML/AI methods replicate patterns in the data by design
If you give it data with human biases, then the AI can easily become biased. This has led to debates about how to use ML (example - ML algos fail to set cash bail without bias)
Amazon’s engineers used ML to evaluate applicants but taught the model that males were automatically better
Criminal sentencing based on “risk predictions” overweight race
Online advertising - Google is more likely to serve up arrest records in searches for names assigned “primarily to black babies”
Humans are strategic and will exploit incentives created by algos and exploit the algo itself
Facebook’s targeted ad categories initially allowed hate groups to form
Microsoft’s chat bot was hijacked by 4chan users to teach the bot hate speech
Uber must consider how changes to its dispatch algorithm will alter driver behavior
ML/AI tools are not always the right tool
Zillow’s pricing model was best-in-class but still lost nearly 400m in a single quarter!
Data leakage is common and can lead to false “discoveries” of impossible performance gains
Some problems are simply hard
Predicting stock returns is hard! The best predictive R2 for individual stocks in this paper (open access here) is just 1.80% per month.
IBM’s Watson tried to predict cancer. How’d it go? According to internal documents: “This product is a piece of sh–.”
Google Flu Trends consistently over-predicted flu prevalence