5.1.1. Machine Learning Gone Wrong¶
But just because you can, doesn’t mean you should.
The classic citation for this argument is from Jurassic Park.
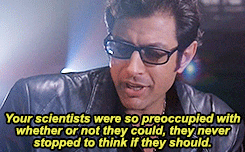
There are many examples of ML applied wrong and practitioners in the space that I talk to spend a lot of time keeping their data science teams from replicating some notable breakdowns:
Google Flu Trends consistently over predicted flu prevalence
IBM’s Watson tried to predict cancer. How’d it go? According to internal documents: “This product is a piece of sh–.”
Amazon’s engineers used ML to evaluate applicants but taught the model that males were automatically better
Chatbots have had many struggles. Here’s Microsoft’s attempt at speaking like the youths:
ML/AI methods replicate patterns in the data by design: If you give it data with human biases, then the AI can easily become biased. This has led to debates about how to use ML for
Criminal sentencing based on “risk predictions” overweight race
Online advertising - Google is more likely to serve up arrest records in searches for names assigned “primarily to black babies”
Google will intelligently stitch together photos, but I guess Google’s AI thought the guy was built like a mountain (congrats, I guess?):
Common problems with analysis (ML or otherwise)
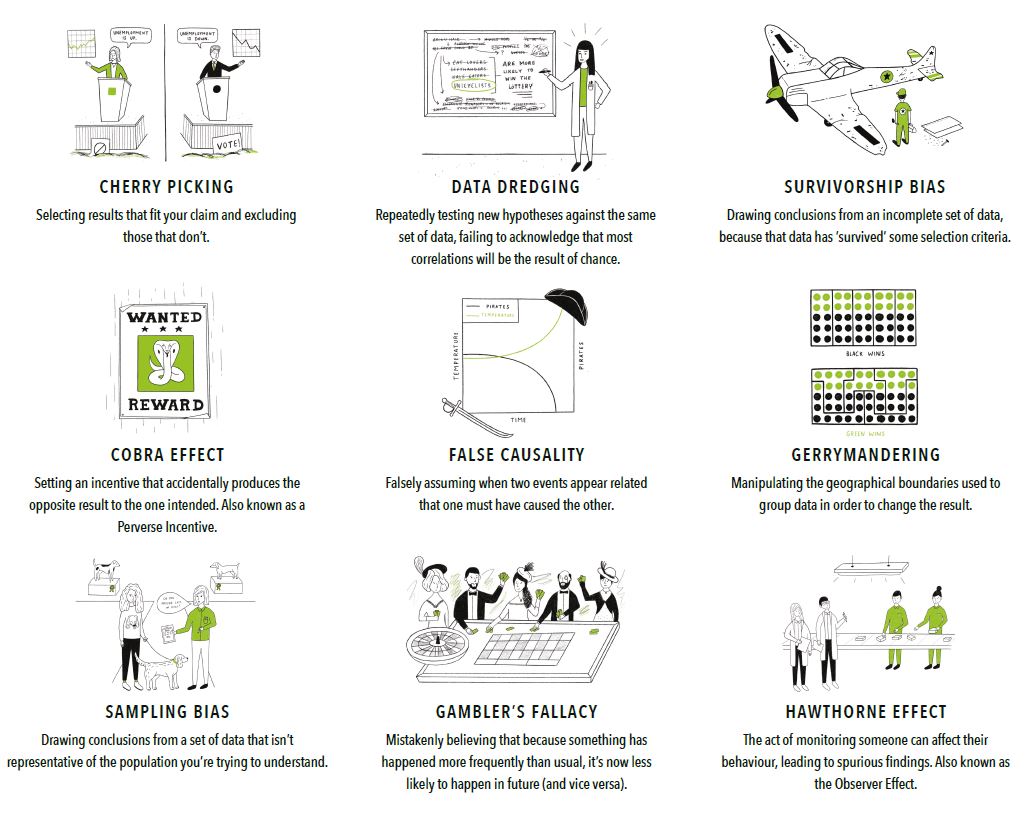
Note
The good news is that these problems can be avoided. Understanding how is something we will defer until we have a better understanding of the methods and processes we will follow in an ML project.